The Future of Deep Learning and Its Impact on AI Innovations
Written on
Chapter 1: The Surge of Deep Learning
In recent years, the field of deep learning has witnessed remarkable growth. Over the last five years, the number of professionals engaged in deep learning has surged from a mere handful to tens of thousands. This surge has been accompanied by a significant acceleration in research activities, showing no signs of deceleration.
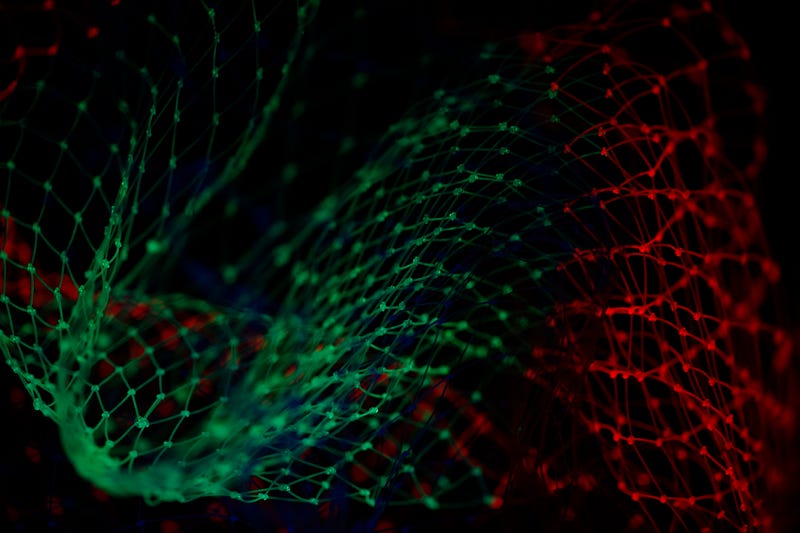
Photo by Pietro Jeng on Unsplash
Self-Supervised Learning
Self-supervised learning represents a pivotal machine learning strategy that enables a model to infer part of its input from another segment. This method, often referred to as predictive or pretext learning, transforms an unsupervised challenge into a supervised one by automatically generating labels. The essence of this approach lies in establishing suitable learning objectives tailored for specific use cases.
As advancements in artificial intelligence persist, self-supervised learning is being harnessed across various domains, including computer vision, cancer detection, and numerous aspects of natural language processing. Its applications extend to sensitive areas, such as assessing content on social media platforms.
Neural Network Architectures Inspired by the Brain
The evolution of deep learning is closely tied to the development of neural network architectures that draw inspiration from the human brain. Researchers are optimistic that these networks can tackle numerous real-world challenges. For instance, one practical use of these networks is in recognizing binary patterns in telecommunication. They are also being explored for solving intricate mathematical equations.
Recent technological innovations have enabled unprecedented observation of neural activities. Techniques like optogenetics allow for the recording of these activities at a single-cell level, while advanced beam-modulation methods facilitate large-scale three-dimensional monitoring of neural interactions. Consequently, the potential for tracking millions of neurons in the future is becoming increasingly feasible.
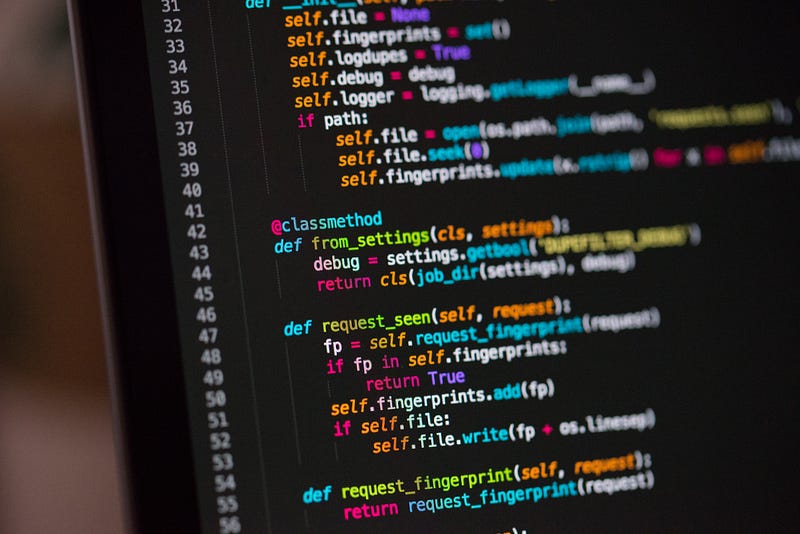
Photo by Chris Ried on Unsplash
Machine Learning as the Next Industrial Revolution
Deep learning, also known as machine learning, is emerging as a transformative force, often likened to the next industrial revolution. Much of this innovation hinges on data—the foundational element for intelligent machines. The proliferation of the internet has made extensive datasets readily accessible. Organizations are now leveraging various data types, including video, imagery, and natural language resources. User-generated image tags have proven to be invaluable for researchers in computer vision.
In the past five years, the community working on deep learning has expanded dramatically, evolving from hundreds to thousands, with research advancements occurring at an unprecedented pace. Investment in this sector continues to thrive, showing no signs of slowing.
Applications in Personalization
Deep learning is a groundbreaking technology designed to emulate brain functions. Its algorithms utilize multiple layers of neural networks to process vast amounts of data and generate predictions. Various applications, including chatbots, self-driving cars, and medical diagnostics, are already benefiting from this technology.
Numerous companies are actively employing deep learning. For example, IBM Watson uses it to analyze player emotions for highlight generation. Similarly, Amazon and Netflix leverage this technology to enhance user experiences through personalized recommendations of shows and films.
A notable manifestation of deep learning's application in personalization is through virtual assistants, which can learn by interpreting natural language. These assistants comprehend spoken language, convert it to text, book appointments, and take notes.
Data Security Challenges
As deep learning systems proliferate, data security has emerged as a crucial concern. The technology's rapid integration into various applications—ranging from piloting autonomous vehicles to mapping crime and diagnosing medical conditions—poses significant risks. For instance, a malicious alteration to an image could mislead a deep neural network (DNN) into misdiagnosing cancer, or a cybercriminal could compromise an online AI system.
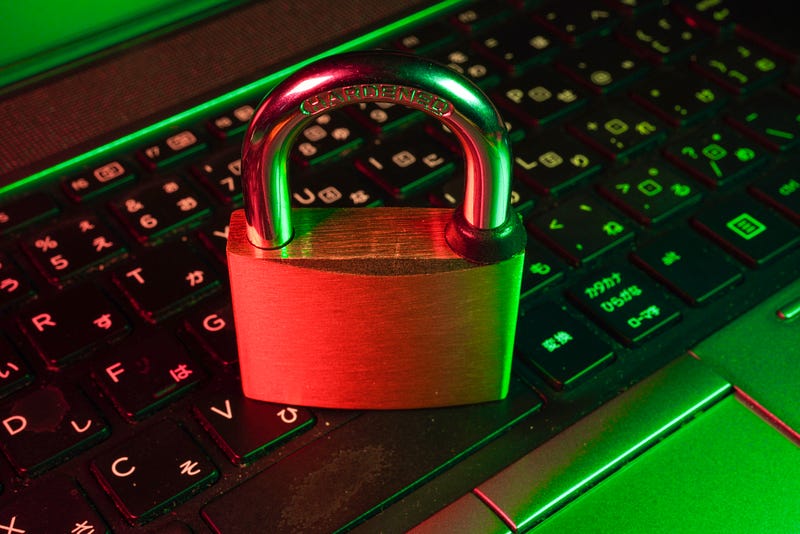
Photo by FLY:D on Unsplash
Chapter 2: The Future of Deep Learning
Exploring the trajectory of deep learning, one can observe the ongoing research and innovations shaping its future landscape.
The first video titled "The Future of Deep Learning" delves into the innovations and future directions of this transformative technology.
The second video, "The AI Pioneers: Geoffrey Hinton, Yann LeCun & Ruslan Salakhutdinov," features discussions from leading figures in AI, shedding light on the advancements in deep learning.