Exploring NeurIPS 2021 Insights and Future Data Trends
Written on
Chapter 1: Overview of NeurIPS 2021
For those who may not be aware, the Neural Information Processing Systems (NeurIPS) conference took place online from December 6 to December 14, 2021. This event is recognized as one of the premier gatherings for machine learning engineers, data scientists, and AI researchers worldwide, serving as a platform for exchanging insights on neural information processing in various contexts—biological, technological, mathematical, and theoretical. As the conference occurs in December, it often provides a glimpse into the anticipated trends in the data science field for the upcoming year.
What data trends can we expect in 2022? Here are some key themes that emerged during NeurIPS this year.
Section 1.1: The Shift Towards Data-Centric AI
The 2021 conference highlighted a notable transition towards a data-centric model of artificial intelligence and machine learning. Attendees observed that simply adjusting algorithms and enhancing hardware is no longer sufficient to develop superior machine learning models. Currently, the primary bottleneck in AI development revolves around data quality, a focus that was prominent throughout the event.
To address this, NeurIPS introduced a new track titled "Datasets and Benchmarks," emphasizing the growing importance of data-centric methodologies in AI. This trend was exemplified by a machine learning competition presented by Andrew Ng, where participants were tasked with optimizing data rather than refining the models themselves.
Section 1.2: Constructing Quality Datasets and Benchmarks
Many presentations also underscored the shift towards prioritizing high-quality datasets. Discussions revolved around the critical nature of data annotation quality, with numerous speakers addressing the challenges associated with attaining superior datasets. Although the community has a solid grasp of how to evaluate model quality, assessing data quality remains largely ambiguous and underexplored.
To clarify this issue, some presenters suggested that measuring errors within a dataset should be regarded as a vital metric for quality assessment. Best practices for data collection were also emphasized, including providing clear instructions, training annotators, and closely monitoring the data gathering process. Such practices are essential for creating datasets, especially when intricate and time-consuming pipelines are involved in data collection and annotation.
Furthermore, the significance of data versioning and documentation emerged as crucial topics, necessary for tracking the evolution of datasets and recording modifications made over time.
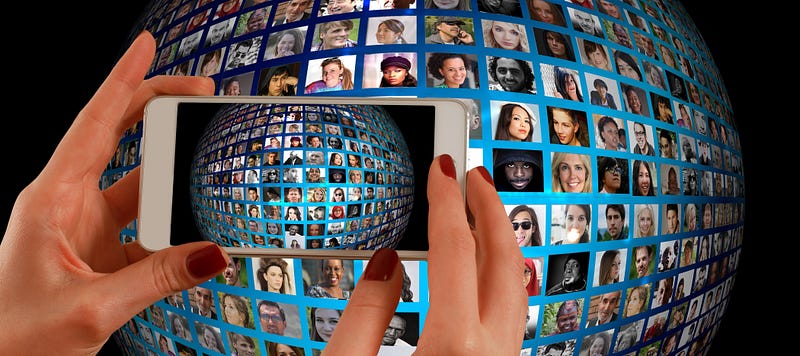
Chapter 2: Addressing Data Ethics
The emphasis on datasets at this year's conference also raised critical discussions regarding data ethics. Many models have been developed using biased datasets, leading to a reflection of those biases in the models’ outcomes. Speakers highlighted the importance of addressing bias during data collection by implementing appropriate sampling techniques and ensuring the inclusion of minority groups, rather than attempting to adjust model parameters later in the process.
The first video titled "Peer Review is still BROKEN! The NeurIPS 2021 Review Experiment (results are in)" discusses the findings from the NeurIPS review process, shedding light on the effectiveness and challenges faced.
Looking Ahead: Future of Data-Centric AI
This article provides a brief overview of significant themes discussed at NeurIPS 2021. Overall, the conference demonstrated a clear pivot towards a data-centric approach in AI, with numerous speakers focusing on data quality and the establishment of best practices in this emerging field.
Looking forward, 2022 appears poised to introduce new metrics for evaluating data quality. It is plausible that measuring data quality may become as standardized as current practices for assessing model quality.
The second video titled "NeurIPS 2023 Poster Session 1 (Tuesday Evening) - YouTube" offers insights into the latest research and innovations presented at NeurIPS, reflecting the ongoing evolution in the field.
Below are additional resources you may find interesting:
- AI-Assisted Annotation with Multimodal Neural Networks
- A real-life example of data validation for an antispoofing face detection project
towardsdatascience.com
- Jupyter Notebook Autocompletion
- The best productivity tool for Data Scientists you should be utilizing.
towardsdatascience.com
- Human-in-the-loop in Machine Translation Systems
- Evaluating machine translation quality using crowdsourcing.
towardsdatascience.com
- Nine tips about Jupyter Notebook you may not be aware of
- Enhance your productivity with these strategies.
towardsdatascience.com