Navigating the Complex Landscape of AI Risks and Progress
Written on
Chapter 1: Understanding AI Risks
The concept of "AI Doomers" suggests that we are on the brink of achieving superintelligence or Artificial General Intelligence (AGI). They argue for a reduction or cessation of AI research based on several beliefs:
- Humanity possesses the capability to create an all-powerful AI.
- There is a risk of accidentally developing such a technology in a rapid advancement scenario, potentially overlooking critical resources like computational power and energy.
- The idea that we can control or align this superintelligence is akin to suggesting that an ant could manage human behavior.
At the same time, Large Language Model (LLM) chatbots continue to exhibit fundamental flaws, such as making basic mathematical errors like dividing by zero. They lack true mathematical understanding, merely imitating patterns that resemble math. Furthermore, they struggle to verify straightforward facts, a significant challenge that remains unsolved, and can fail dramatically when faced with tasks that extend beyond their training data.
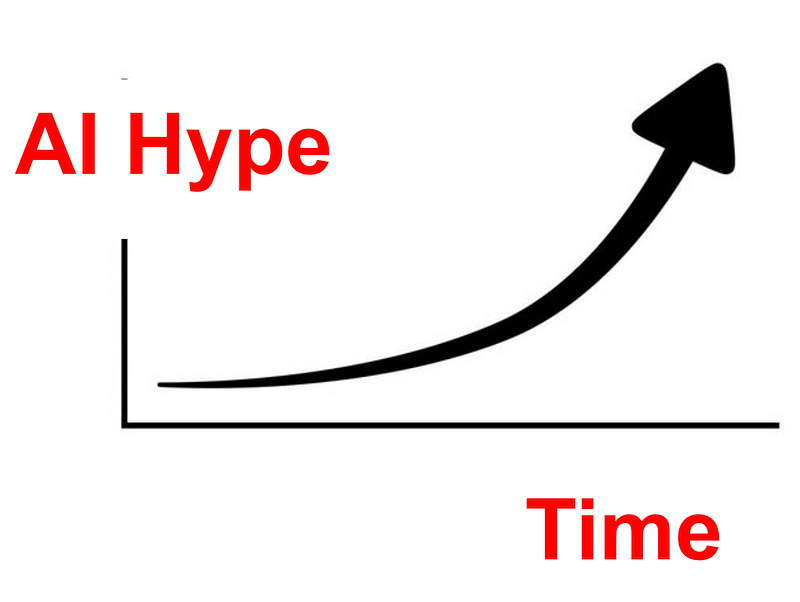
The exponential growth in AI hype often leads to misconceptions about the capabilities of LLMs. They may seem intelligent because they produce human-like responses, but this is misleading.
The analogy of constructing a ladder to the moon in a pre-modern society aptly illustrates the hurdles we face in achieving superintelligence. While it might seem achievable by merely adding more rungs, significant scientific breakthroughs and engineering challenges must be addressed. Many of these concepts, such as Newtonian physics and rocket technology, were unknown to those civilizations.
My concern lies in the stagnation of scientific advancement and the potential for another AI winter. While humanity's technological progress may appear to follow a single exponential curve, it is more accurately represented by overlapping S-curves. LLMs are remarkable, yet they do not possess true reasoning abilities, and we require additional scientific breakthroughs to reach superintelligence.
The pressing question remains: why hasn't AI resolved critical global issues like nuclear conflict, cancer, heart disease, or energy crises? The answer lies in the fact that these challenges extend beyond the training data and necessitate innovative, complex thinking.
Although scenarios of rapid self-improving superintelligence as suggested by thinkers like Tegmark, Yudkowsky, and Bostrom are theoretically possible, we must also be cautious of more immediate concerns.
For insights on the current pace of scientific progress, consider the work of Donald and Stuart Geman in "Science in the Age of Selfies." These scholars highlight a troubling slowdown in scientific advancement. I contend that the developments in LLMs and AI should be viewed within this context. The technological leap we experienced with GPUs revitalized AI research, but many foundational ideas, such as deep neural networks, have existed for decades.
This discussion responds to recent conversations surrounding AI's hype cycle, particularly the resignation of employees at OpenAI who felt the organization was not doing enough to address the alignment of superintelligence. We currently face pressing AI safety and ethical issues, including mental health, discrimination, fraud, and the misuse of technology by authoritarian regimes.
Chapter 2: The ARC Prize and the Quest for True Reasoning
The first video, "The Age of AI: Myths, Risks, and Opportunities," delves into the misconceptions surrounding AI's capabilities and the existential risks posed by advanced technologies.
The second video, "AI Progress SLOWING DOWN! Bad News for E/ACC, Good News for SAFETY! Let's Unpack All This!" discusses the implications of slowing AI advancements and the potential benefits for safety and ethical considerations.
The ARC Prize represents a significant initiative, offering over $1 million to develop an AI system capable of genuine reasoning. Established by François Chollet of Google, who created the Keras framework for TensorFlow, this competition seeks innovative solutions to the ARC-AGI benchmark.
Participants can engage with the challenges, which may appear straightforward, yet no AI system has successfully met them due to the requirement for reasoning, as opposed to mere regurgitation of training data. This challenge extends to the latest models from OpenAI, Anthropic, and Meta, which require substantial investments for training.
Ultimately, current LLMs resemble advanced search engines that excel in language processing, progressively improving as more information is indexed. What is often perceived as reasoning or thought is, in reality, an illusion rooted in language, the primary medium of human communication. Moreover, LLMs are nearing a performance plateau as a function of data, indicating that exponentially more data is needed to achieve only marginal gains in performance.
(Note: I have not been regularly posting on Medium for some time, hindered by the challenge of crafting polished drafts. This piece represents an effort to share something quickly and will undergo future refinements. The priority is to disseminate ideas rather than perfect them; after all, perfection can hinder progress.)